What the Cal is Continuous Active Learning? And Why Should the Trial Lawyer Care.
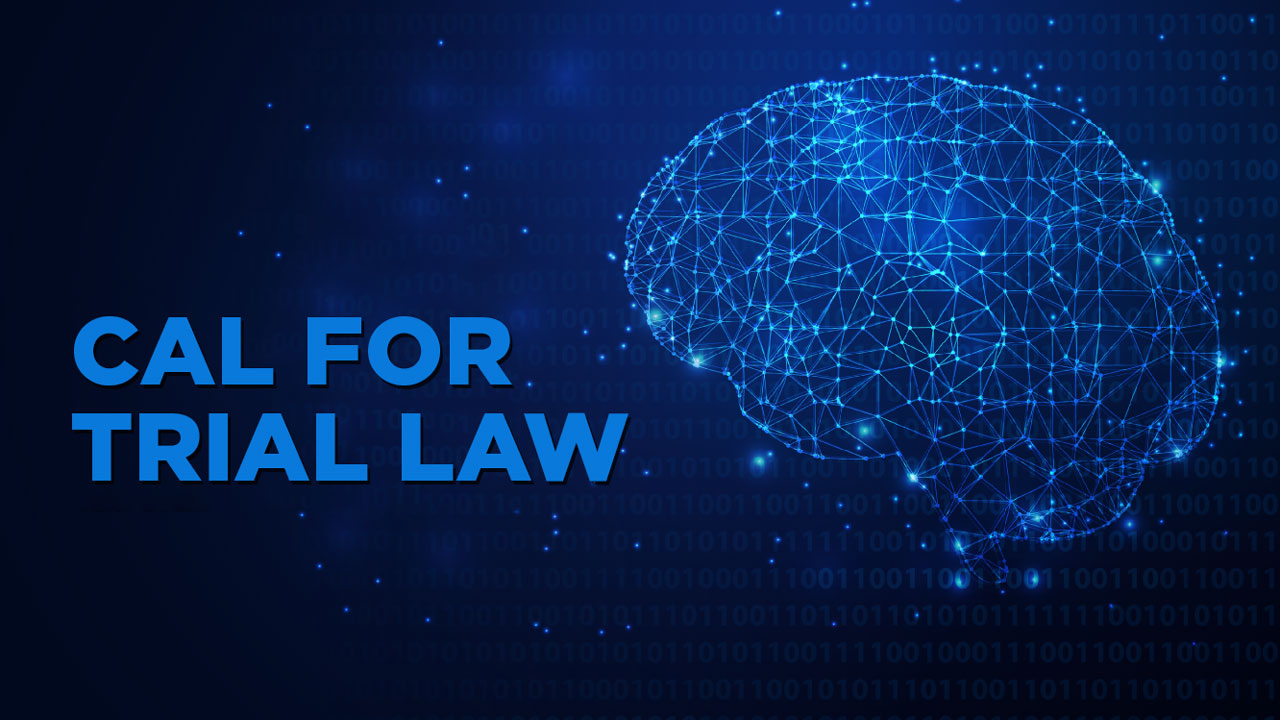
Continuous Active Learning, or CAL, is the term e-discovery computer science techies use when referring to the next generation of Technology Assisted Review tool—aka TAR 2.0—in matters of litigation. It saves a bunch of time and money, delivering document discovery results in real-time, a distinct advantage over TAR 1.0, predictive coding model. So, for practitioners looking to increase the speed and accuracy of their document review process, you should care.
What’s Wrong with Predictive Coding?
Well, nothing, generally speaking. For more than a decade now, TAR 1.0 has proven that technology-assisted review is more efficient and accurate than human review alone. But there are some limitations that come with using predictive coding models.
Put simply, TAR workflow is an iterative process that includes multiple steps:
- Subject matter expert (SME) creates a set of sample documents known as a seed set that “trains” the software to find other similar documents.
- SME codes the documents “relevant” or “irrelevant”.
- SME inputs the documents into the software.
- The software applies coding to the documents and draws inferences.
- The software then ranks the documents in order of likely relevance.
- SME verifies if the software is accurately identifying the documents and adds more coding if necessary.
- The software creates its own algorithms for reviewing and identifying document relevance.
- SME repeats the process several times until you get the accuracy levels you are looking for. This is because the seed sets typically include documents with low richness or values.
Sure, TAR 1.0 is an alternative to the manual review of e-discovery documents, but if you could simplify the process even more, wouldn’t you?
Enter TAR 2.0 or CAL
CAL takes a non-iterative approach. It uses an Artificial Intelligence (AI) engine to actively learn and identify the most relevant information in your documents with real-time updates to relevance scoring and prioritization based on targeted human input. And it only gets better with each new batch of data.
Think of CAL like a music streaming service that “chooses” which songs you might like to hear based on your previous selections. In the eDiscovery workflow process, users review and tag a small portion of the data set which is used to train the software. The algorithm learns in the background, analyzing the tags and develops a logical method of ranking the data from most to least relevant. A model is built for each tag, and as each new document is tagged, the model gets more accurate for each tag. After a model reaches a certain quality measure, it can be applied to the entire data set. No seed sets to evaluate.
The iCONECT AI Difference
At JURIS LTS, we utilize the powerful iCONECT AI with Continuous Active Learning for litigation eDiscovery and case data review. It’s faster than any other tool on the market because it allows for the review of multiple issues in a single pass, and can handle all types of data, including email and office files. In addition, iCONECT’s prediction engine ranks the entire information set together, rather than a limited number of randomly selected documents. New documents added to the population simply join the ongoing ranking process. After the model is set, it can be applied to any additional documents to generate further results ‘with one click’. When you use a tool that lets you zero-in on the most relevant information, it:
- Reduces the number of documents to review.
- Reduces the time spent reviewing irrelevant documents.
- Reduces the amount of human time needed to review.
- Reduces billable review time to clients.
Access CAL technology to improve outcomes, and reduce time and expenses associated with discovery and trial and arbitration prep. For more information, visit us online or call us at (888) 991-8108.